If you’re lucky enough to live in a community with access to fresh drinking water, you may be guilty of taking this life-giving substance for granted. Under normal circumstances, you probably don’t give much thought to the many steps it takes to get that clean water to your kitchen faucet. It’s not until a pipe bursts, a major storm knocks out power to a processing plant, or lead contamination is discovered that your water supply becomes front and center. Suddenly, you’re on the phone with your water utility asking what went wrong and when service will be restored.
Where are we going with this? Having an awareness and appreciation of the work these utilities perform isn’t just an exercise in gratitude. It can also help us make sense of the role, value, and “fluidity” of data throughout an organization. In many ways, data analytics is like your water utility. We rely on our local water utility to capture water from many different sources, treat it, and distribute it. Likewise, we expect data analytics tools, such as NI’s SystemLink™ and OptimalPlus (O+) software, to grab data from many sources and make it accessible to us to draw value from it.
STEP 01: Grab the Water
We can easily imagine all the different types of water the utility manages: rainwater, sewage, natural sources, and so on. Depending on that type, different processing would be needed to avoid polluting the water by mixing it prematurely. Once the water is caught properly, it’s brought to processing facilities. Like water, data is inconsistent, everywhere, and usually fragmented. Think databases, CSV files, MESs, hard drives, cloud services, data lakes, and anywhere data is stored. We don’t want to pollute or mix the data without preparing it, so we use data analytics software on all our data sources for pulling the data together in a single, accessible, contiguous source that is independent from the original data sources and raw formatting.
STEP 02: Clean the Water
Sewage water would certainly require more treatment than water from natural springs, so it takes longer to mix them together and purify them to a standard level. After data has been pulled together, data analytics software processes it to translate it into a single, common, consistent source. As part of this, some data that’s not numerical runs through a parser to make it numerical, for example, when the data is a photo of a radar module assembly, or a snapshot frame from an advanced driver assistance systems (ADAS) camera.
STEP 03: Deliver the Water to the Faucet
Once the water is purified, it must be distributed with enough pressure from the plant to the places it needs to be: from water tanks, underground pipelines, and in-house pipes all the way to a faucet, shower head, or washing machine. After the data analytics software has prepared or “purified” the data by making it consistent, keeping it together, and correlating it properly, the fun part starts: delivering it to the source where it’s needed. In business terms, this means impacting the bottom line through the insights that come from the data. We recognize how that “bottom line” changes. Just like a faucet represents a different use case than a shower head for the same water, a process engineer needs something different from the test engineer, or the sustaining group. NI’s data analytics software automatically delivers the data that each of them needs, to the outlet where they need it, so they can detect problems faster.
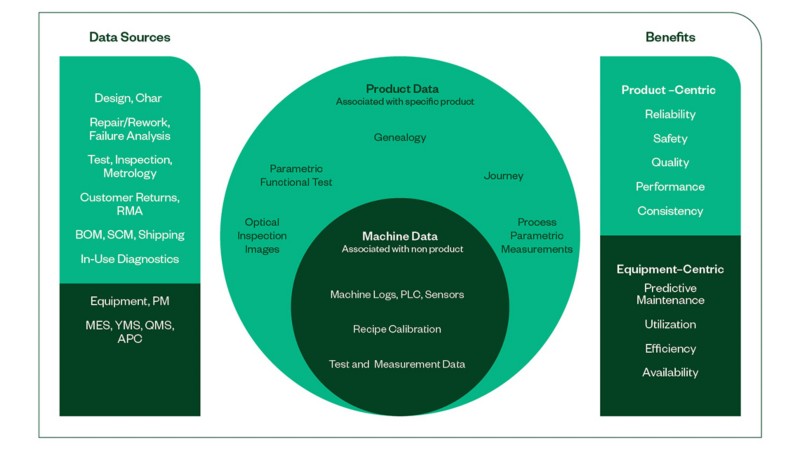
NI’s product-centric approach offers advanced data analytics in real time.
What Does This All Mean?
As an example, modern cars are increasingly equipped with radar sensors for safety functions like autonomous emergency braking or blind spot monitoring. One of the tests that those radars undergo assesses their ability to determine an object’s distance precisely. Let’s say we expect the measured distance from the radar under test to follow a Gaussian curve with the center at 25 m, and all variation in a distribution to stay within a couple of centimeters. To make sure this happens, we sample results every few days, look at the data, and make sure the curve is plotting as expected. If something fails and the distribution moves, we pay a cost associated with retesting, reworking, downtime of the test equipment, and so on. The cost depends on the root cause, but we’ve lost time regardless. With NI’s data analytics software, we can automatically run that analysis every few minutes and immediately alert the right people of any problem such as the skewing of that curve. By raising this alert at the right moment, we can act sooner and plan better for whatever downtime we need. In this example, members of the test engineering group defined the measured distance plot as their “faucet,” or their use case. Just like a shower and washing machine are connected to different faucets, other or multiple data “faucets” may be programmed from all the available product, process, supply chain, and test equipment data to provide the following in real time:
- Scrap
- Yield
- Test time
- Asset utilization
- Process efficiency and capacity
- Adaptive manufacturing
- Artificial intelligence and machine learning enablement
- Predictive maintenance
In other words, you receive insights about your product and the test equipment in real time with NI’s data analytics software. NI’s product-centric approach offers advanced data analytics in real time.
Closer to Your Vision
Whether you’re developing autonomous vehicles, silicon wafers, or defense drones, conducting smarter test and smarter manufacturing through data analytics has a positive impact on all relevant variables, including time to market, time to results, total cost of ownership, risk reduction, and liability reduction. Though this is not the only technology that will get us there, NI strongly believes that through the right analysis of impact and commitment to change, implementing the NI data analytics solution is not a gamble on data analytics. It’s a solid step toward making data work for you to drive insights.